The revolutionary results of Deep Learning (DL, referred to as DL 1.0) are ubiquitous around us. Underlying this progress is a huge human effort to find the right working conditions through expert intuition and a large number of trials and errors. AutoML originally aimed at taking humans out of the loop by relieving them of the overhead of tuning machine learning models for their tasks. However, under the modern context of large-scale DL that is finding applications in newer domains every day, it is both essential to tune the DL models better and to do so efficiently under tractable compute budgets.
DL 2.0 revisions the practice of DL, with the help of a human-centered AutoML to unify the training pipeline of DL 1.0 by tuning the pipeline as well, tailored for the specific requirements.
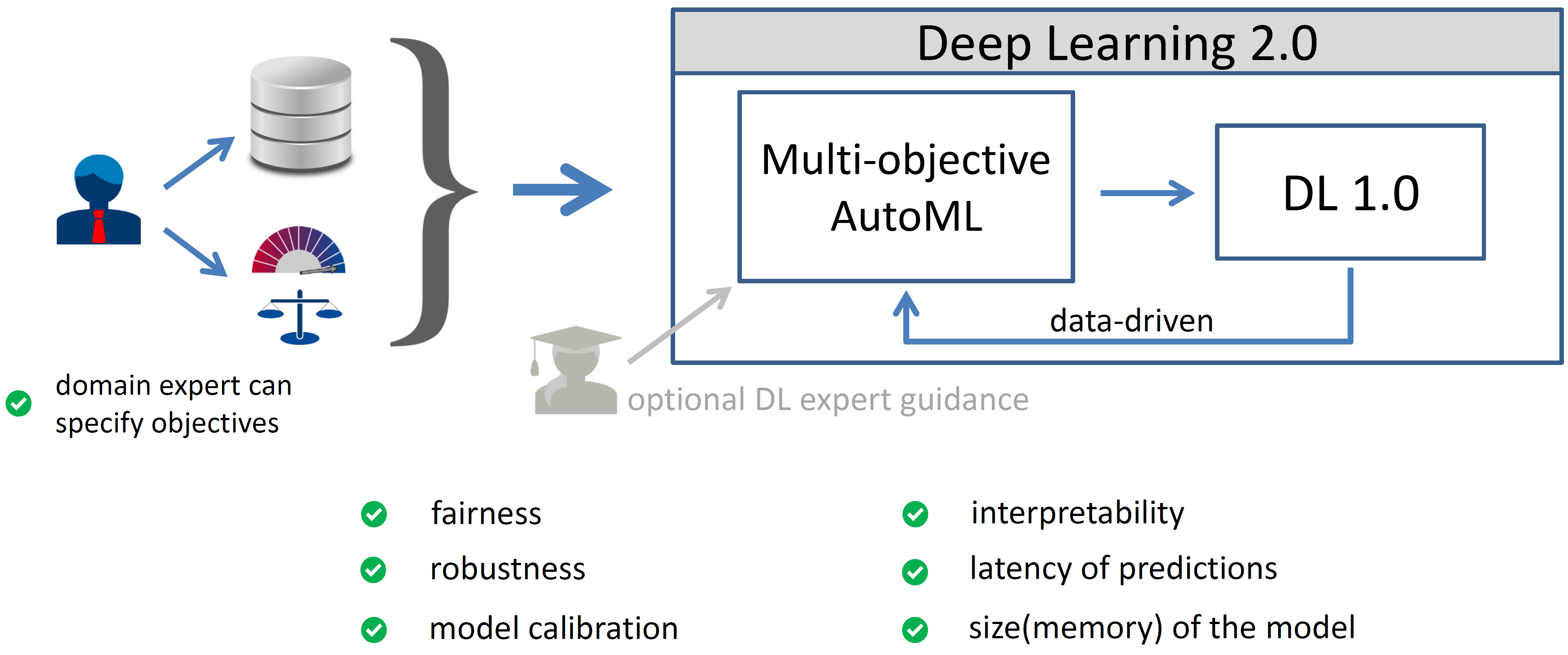
More details on Deep Learning 2.0 can be found in this blog post by Frank. Hutter.