By Compared to the state of computer vision 20 years ago, deep learning has enabled more generic methodologies that can be applied to various tasks by automatically extracting meaningful features from the data. However, in practice those methodologies are not as generic as it looks at first glance. While standard neural networks may lead to […]
AutoDispNet: Improving Disparity Estimation with AutoML
Posted on August 30, 2019 by Arber Zela, Yassine Marrakchi, Frank Hutter
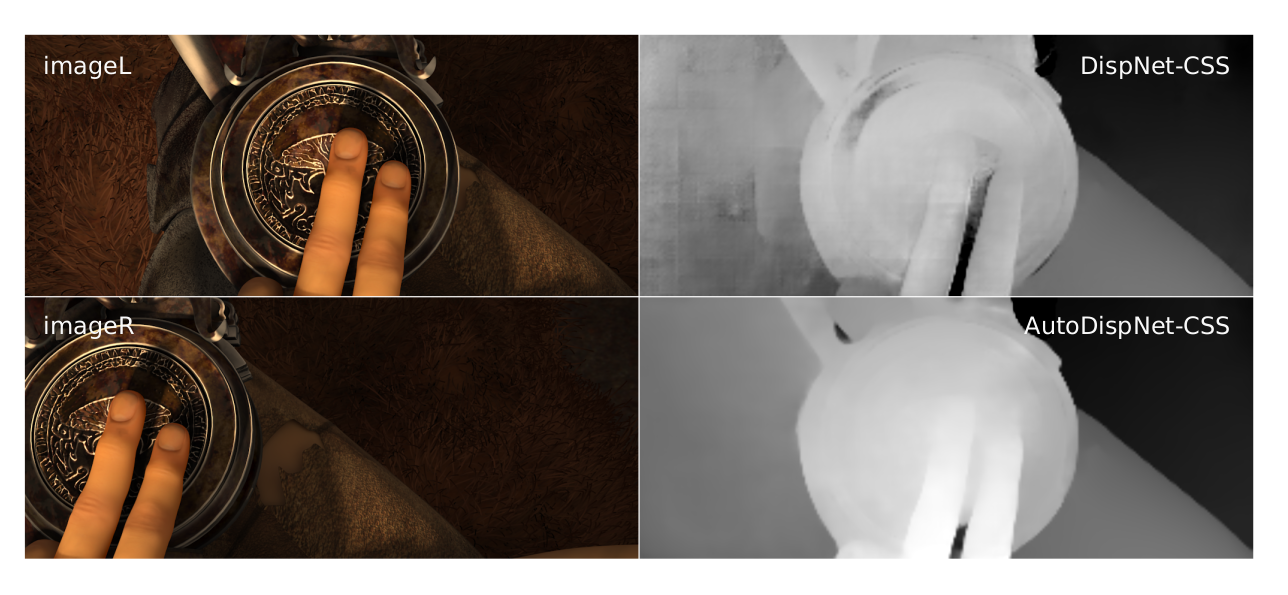